A new definition of “probability”
from Asad Zaman
An article by Alan Hajek in Stanford Encyclopedia of Philosophy lists six major categories of definitions. Many more are possible if causality is also taken into account. These definitions conflict with each other, and face serious problems as interpretations of real-world probabilities. The basic definition of probability we will offer in this lecture falls outside all of these listed categories. Before going on to present it, we briefly explain why there is such massive confusion about how to define probability. read more
Leave a comment Cancel reply
This site uses Akismet to reduce spam. Learn how your comment data is processed.
Real-World Economics Review
WEA Books
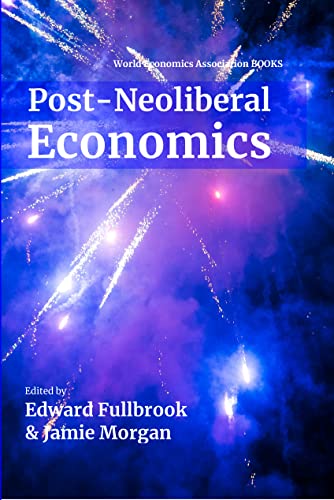
follow this blog on Twitter
Top Posts- last 48 hours
- Lost opportunities?
- The problem with electric vehicles
- There ain’t no libertarians, just politicians who want to give all the money to the rich
- Weekend read - A STIGLITZ ERROR?
- Economics — a dismal and harmful science
- With a modest financial transactions tax, Jim Simons would not have been superrich
- Comments on RWER issue no. 69
- Distribution and redistribution of wealth in USA
- Econometrics and the art of putting the rabbit in the hat
- comments on RWER issue no 76
"We cannot solve our problems with the same thinking we used when we created them." Albert Einstein
Regular Contributors
Real World Economics Review
The RWER is a free open-access journal, but with access to the current issue restricted to its 25,952 subscribers (07/12/16). Subscriptions are free. Over one million full-text copies of RWER papers are downloaded per year.
WEA online conference: Trade Wars after Coronavirus
Comments on recent RWER issues
————– WEA Paperbacks ————– ———– available at low prices ———– ————- on most Amazons ————-
WEA Periodicals
----- World Economics Association ----- founded 2011 – today 13,800 members
Recent Comments
- David Harold Chester on Weekend read – A STIGLITZ ERROR?
- David Harold Chester on Weekend read – A STIGLITZ ERROR?
- sackergeoff on With a modest financial transactions tax, Jim Simons would not have been superrich
- CBASILOVECCHIO on Weekend read – A STIGLITZ ERROR?
- David Harold Chester on Weekend read – A STIGLITZ ERROR?
- pfeffertag on Weekend read – A STIGLITZ ERROR?
- CBASILOVECCHIO on Weekend read – A STIGLITZ ERROR?
- Arbo on Economics — a dismal and harmful science
- spamletblog on Economics — a dismal and harmful science
- bckcdb on Economics — a dismal and harmful science
- David Harold Chester on Real-world economists take note!
- Patrick Newman on Real-world economists take note!
- deshoebox on Real-world economists take note!
- felipefrs on The non-existence of economic laws
- Seeker on The non-existence of economic laws
Comments on issue 74 - repaired
Comments on RWER issues
WEA Online Conferences
—- More WEA Paperbacks —-
———— Armando Ochangco ———-
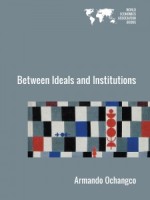
Shimshon Bichler / Jonathan Nitzan
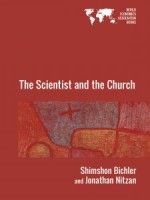
————— Herman Daly —————-

————— Asad Zaman —————
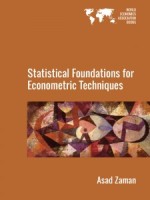
—————– C. T. Kurien —————
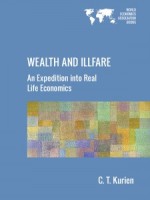
————— Robert Locke —————-

Guidelines for Comments
• This blog is renowned for its high level of comment discussion. These guidelines exist to further that reputation.
• Engage with the arguments of the post and of your fellow discussants.
• Try not to flood discussion threads with only your comments.
• Do not post slight variations of the same comment under multiple posts.
• Show your fellow discussants the same courtesy you would if you were sitting around a table with them.
Most downloaded RWER papers
- What Is Neoclassical Economics? (Christian Arnsperger and Yanis Varoufakis)
- The housing bubble and the financial crisis (Dean Baker)
- Global finance in crisis (Jacques Sapir)
- The state of China’s economy 2009 (James Angresano)
- Green capitalism: the god that failed (Richard Smith)
- New thinking on poverty (Paul Shaffer)
- Debunking the theory of the firm—a chronology (Steve Keen and Russell Standish)
- Why some countries are poor and some rich: a non-Eurocentric view (Deniz Kellecioglu)
- Trade and inequality: The role of economists (Dean Baker)
Family Links
Contact
follow this blog on Twitter
RWER Board of Editors
Nicola Acocella (Italy, University of Rome) Robert Costanza (USA, Portland State University) Wolfgang Drechsler ( Estonia, Tallinn University of Technology) Kevin Gallagher (USA, Boston University) Jo Marie Griesgraber (USA, New Rules for Global Finance Coalition) Bernard Guerrien (France, Université Paris 1 Panthéon-Sorbonne) Michael Hudson (USA, University of Missouri at Kansas City) Frederic S. Lee (USA, University of Missouri at Kansas City) Anne Mayhew (USA, University of Tennessee) Gustavo Marqués (Argentina, Universidad de Buenos Aires) Julie A. Nelson (USA, University of Massachusetts, Boston) Paul Ormerod (UK, Volterra Consulting) Richard Parker (USA, Harvard University) Ann Pettifor (UK, Policy Research in Macroeconomics) Alicia Puyana (Mexico, Latin American School of Social Sciences) Jacques Sapir (France, École des hautes études en sciences socials) Peter Söderbaum (Sweden, School of Sustainable Development of Society and Technology) Peter Radford (USA, The Radford Free Press) David Ruccio (USA, Notre Dame University) Immanuel Wallerstein (USA, Yale University)
Interesting but a bit too inspired by “physics”, both in the claim that the classical idea of probability was based on laplacian mechanicism, and in the idea of probability based on “forking paths”/realities.
The concepts of probability that I personally like, those based on information theory and on Finetti’s (operational, subjectivist) “betting” based concepts should have been given some more attention.
However in particular I am quite perplexed by the illustration of the bayesian approach given using a sequence of single events. To my understanding that is quite objectionable: the Bayes formula in itself is just a formula, there is no need to interpret it.
The interpretation is needed to justify doing bayesian inference from measures on sample to measures of the the population, and to do that is not complicated: applying a prior is a subjective bet that “we” know that the sampling process is biased in a specific way for which correction is needed in order to reveal the “true” measures of the population, or at least improve the signal-to-noise ratio.
That does not depend on whether the sampling is “ensemble” (“cross-sectional”, “spacewise”) or “ergodic” (“logitudinal”, “timewise”), the question of ergodicity and “causality” and time in probability are not directly related to that. Noise arises in both cases, because of sampling.
In general though I am not comfortable with the concept of probability of single events, for me “probability” makes sense only for samples of ergodic (not in the sense used by Ole Peters even if it is related) sources, as in the information theory based approach. I have found that NN Taleb has reached much the same conclusions (probably because we share part of our sources), for example:
“Entropy (Informational) is the way “evidence-based” science should go.”
“The title is blown up but the article is right on point. You miss on ergodicity, you get nothing in decision-making under uncertainty.”
“redictions must have #skininthegame hence MUST be subjected to arbitrage boundaries. ‘An options-pricing approach to election prediction”
As an aside, a point that was drilled in me by my amazing professor of statistics and probability and it seems rarely taught elsewhere, is that “statistics” means two completely different things:
* Statistics involving “regular numbers”: these are statistics on populations, e.g. “the average of 2 and 6”, and “probability” is not involved in any way.
* Statistics involving “stochastic numbers”: these are statistics on samples when *inferred* (which is a bet) to represent the statistics of the population.
Note: the statistics on a sample are “regular numbers”, with respect to the sample, they become “stochastic numbers” *only* when used to infer the statistics of the population.
These points are very important for political economy studies: the statistics we get (e.g. time series) are *samples* and through them we aim to infer the true statistics of the population, that is those of the “true” model of every political economy.
JM Keynes, in his criticism (sometimes mentioned in this blog) of Tinbergen’s approach, was sceptical about such inference because he reckoned that the samples were not from an ergodic (in these sense of information theory) source, because the “true” population, the structure of the economy, changes fast enough that we cannot get samples large enough (with a low enough signal-to-noise ratio) that they are much useful.
A paradox arises when you try to analyse economic data. Most economic theories take a ceteris paribus form because an economy is embedded in society and subject to all sorts of influences, political cultural etc. If there are any valid generalisations in economics, therefore, you would expect them to become apparent only over an extended number of observations, giving all the other factors time to “average out”. No theory of interest rates or exchange rates, for example, even pretends to explain the high-frequency movements of these variables because they depend on fluctuating sentiment and the accident of market positions. Economies, however, are evolving. Tastes, products and structures are changing continually which is highly likely to change any relationship under consideration. Certainly any particular parameterization of a relationship will change. Is there a gap, or rather a window of opportunity, between the noisy short-term and the ever-evolving long term, in which an economic relation can be tested or measured? There is no guarantee but unless we look for one economics becomes a branch of theology.
The interesting thing about Bayesian methods is that any controversy about them is the province of philosophers not practitioners. When the armed services of any country look for a crashed aeroplane site they combine fragments of probabilistic information using a Bayesian approach. They do so because it works better than any other method tried to date. Bayesian stats are used because they work. Note that the site of the crash is certain in the Asad sense but so what? We still have to find it. People bet on past events as easily as on future events.
Probability is always a matter of epistemology; nature may be entirely deterministic for all we know. Take out human observers and there is no such thing as objective probability.
If you think you know anything, as more evidence comes in Bayes tells you how to use it to update what you know. If you turn out to be wrong the fault was in your prior belief, not Bayes. Or should I say not Price. It was my compatriot Richard Price who extracted Bayes law from the notebooks of his deceased friend Thomas Bayes. Both were non-conformist Christian ministers, Bayes a Presbyterian from London and Price a unitarian from Llangeinor in Wales.